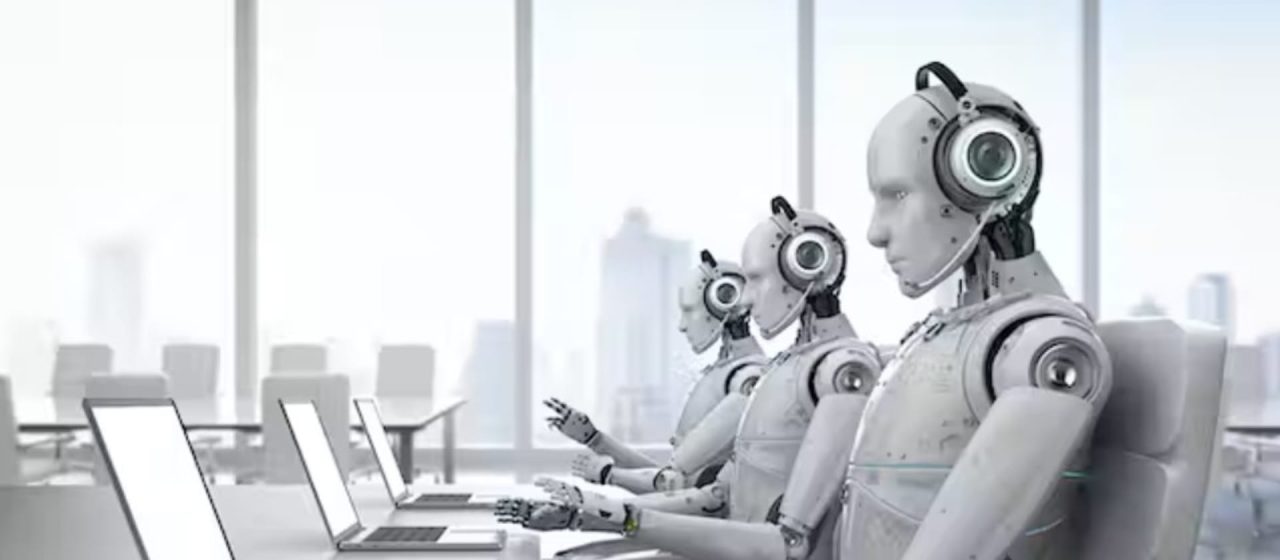
Click here to listen to the interview.
Mark Finlayson, Associate Professor of Computer Science, Florida International University
Large language models are sophisticated sequence completion machines: Give one a sequence of words (“I would like to eat an …”) and it will return likely completions (“… apple.”). Large language models like ChatGPT that have been trained on record-breaking numbers of words (trillions) have surprised many, including many AI researchers, with how realistic, extensive, flexible and context-sensitive their completions are.
Like any powerful new technology that automates a skill – in this case, the generation of coherent, albeit somewhat generic, text – it will affect those who offer that skill in the marketplace. To conceive of what might happen, it is useful to recall the impact of the introduction of word processing programs in the early 1980s. Certain jobs like typist almost completely disappeared. But, on the upside, anyone with a personal computer was able to generate well-typeset documents with ease, broadly increasing productivity.
Further, new jobs and skills appeared that were previously unimagined, like the oft-included resume item MS Office. And the market for high-end document production remained, becoming much more capable, sophisticated and specialized.
I think this same pattern will almost certainly hold for large language models: There will no longer be a need for you to ask other people to draft coherent, generic text. On the other hand, large language models will enable new ways of working, and also lead to new and as yet unimagined jobs.
To see this, consider just three aspects where large language models fall short. First, it can take quite a bit of (human) cleverness to craft a prompt that gets the desired output. Minor changes in the prompt can result in a major change in the output.
Second, large language models can generate inappropriate or nonsensical output without warning.
Third, as far as AI researchers can tell, large language models have no abstract, general understanding of what is true or false, if something is right or wrong, and what is just common sense. Notably, they cannot do relatively simple math. This means that their output can unexpectedly be misleading, biased, logically faulty or just plain false.
These failings are opportunities for creative and knowledge workers. For much content creation, even for general audiences, people will still need the judgment of human creative and knowledge workers to prompt, guide, collate, curate, edit and especially augment machines’ output. Many types of specialized and highly technical language will remain out of reach of machines for the foreseeable future. And there will be new types of work – for example, those who will make a business out of fine-tuning in-house large language models to generate certain specialized types of text to serve particular markets.
In sum, although large language models certainly portend disruption for creative and knowledge workers, there are still many valuable opportunities in the offing for those willing to adapt to and integrate these powerful new tools.
This is an excerpt from the full article, which can be found at news.fiu.edu.