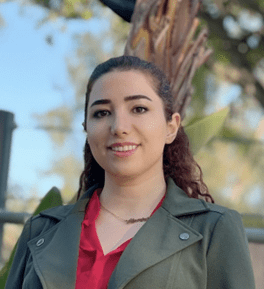
Yasaman Saadati
Florida International University
Lecture Information
CASE-349 & Zoom
2024-06-12 14:00:00
Abstract
Federated Learning (FL) is a decentralized machine learning (ML) technique that enables collaborative training of ML models on distributed devices while preserving data privacy. However, heterogeneity in data, devices, and models deteriorates the performance and scalability of FL. Personalized FL (PFL) addresses this issue by training personalized local models for each client while collaborating towards a shared global model. This Proposal aims to develop robust and efficient PFL approaches for improved generalization and personalization accuracy. Specifically, this research investigates three main research questions: 1) how to optimize hyperparameters in PFL settings?, 2) how to enhance the accuracy and address catastrophic forgetting in PFL algorithms?, and 3) how to leverage meta-learning, transfer learning, and multi-task learning for personalization in PFL? Also, novel solutions are proposed to address this questions including: 1) Auto-FL, an automated hyperparameter optimization approach for FL that integrates local feedback from clients to accelerate the tuning process, 2) pMixFed, a client-adaptive mixup approach for enhanced generalization and personalized accuracy, and 3) A Dynamic approach for adjusting parameter sharing in partial PFL models in both broadcasting and aggregation stages to alleviate the out-of-sample and participation gaps. The preliminary experiments show the effectiveness of the proposed methods in terms of accuracy, generalization, and efficiency compared to traditional FL approaches. This Proposal contributes to the advancement of PFL by addressing critical challenges and providing practical solutions for deployment in real-world applications.
Biography
Yasaman Saadati is a Ph.D. Candidate in Knight Foundation School of Computing and Information Sciences, Florida International University (FIU). She is a member of Sustainability, Optimization, and Learning for InterDependent networks laboratory (solid lab) and working under the supervision of Dr. M. Hadi Amini. Her research interests span Federated Learning, Meta Learning, Multi–Task Learning, and their applications. Yasaman received her M.Sc. in AI from the Iran University of Science and Technology and her B.S. in Computer Engineering from Shahid Beheshti University. Prior, joining FIU, Yasaman also worked as a Data Engineer for two years. She has conducted research on Hyper-parameter optimization in federated learning setting, reinforcement learning algorithms for flow solver optimization, and super-resolution techniques for rapid high fidelity flood inundation map generation. She has published several articles including ICAI’22 and Journal of Hydrology. Yasaman is also a recipient of several awards, including the CRA NSF conference scholarship, CVPR and Grace Hopper travel grant, and the Richard Tapia poster award.