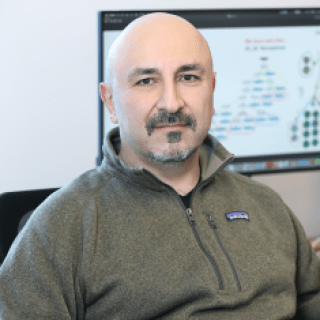
Dr. S. Cenk Sahinalp
Senior Investigator, Head, Tumor Evolution and Genetics Section, Cancer Data Science Laboratory, CCR, NCI, NIH
Lecture Information
CASE 241
2024-04-19 13:00:00
Abstract
Cancer evolution leads to intratumor heterogeneity (ITH), making treatment and cure challenging. In this talk we will demonstrate that it is possible to trace subclonal evolution of a tumor by using full transcriptome single-cell RNA sequencing (scRNAseq) using expressed mutations. For overcoming key challenges involved in the mutational analysis of scRNAseq data such as scale and noise we will introduce Trisicell (https://trisicell.rtfd.io), a powerful computational toolkit designed to infer tumor progression history, assess the resulting tree’s reliability, and investigate the implied genetic and non-genetic ITH.
To test Trisicell, we generated matched bulk DNA, RNA and scRNAseq datasets from preclinical melanoma studies involving immune checkpoint blockade (ICB) therapy. These datasets provide a “gold standard” resource for investigating tumor lineage tracing, ITH and response to immunotherapy for future studies. The progression histories inferred through mutations observed in these matched DNA, RNA and scRNAseq datasets were highly concordant, strongly suggesting that expressed mutations in scRNAseq data enable accurate reconstruction of tumor progression histories. Furthermore, these and other tumor progression trees inferred by Trisicell for clinical studies of ICB reveal that the clonality and subcellular location of neoantigens derived from frameshift mutations are critical drivers of ICB response. Trisicell inferred trees also suggest that major subclones develop distinct adaptive mechanisms to cope with ICB, indicating intratumor heterogeneity of therapeutic resistance. Trisicell and our datasets provide important resources for tracing the subclonal evolution of neoantigens at single-cell resolution, with implications for understanding melanoma evolution and identifying targets for immunotherapy, including ICB and cancer vaccines.
Biography
S. Cenk Sahinalp received his B.Sc. in Electrical Engineering at Bilkent University, Ankara, Turkey, and his Ph.D. in Computer Science from the University of Maryland, College Park. His Ph.D. thesis introduced the first work on the optimal parallel algorithm for suffix tree construction and the first linear time algorithm for pattern matching. After a brief postdoctoral fellowship at Bell Labs, Murray Hill, he has worked as a Computer Science professor, most recently at Indiana University, Bloomington.
Sahinalp’s research has focused on combinatorial algorithms and data structures, primarily for strings/sequences, and their applications to biomolecular sequence analysis, especially in the context of cancer. In the past decade, his lab has developed several algorithmic methods for efficient and effective use of high-throughput sequencing data for better characterization of the structure, evolution, and heterogeneity of cancer genomes. He has (co)trained more than two dozen Ph.D. students and postdocs - many of them now hold independent academic and research positions in the U.S., Canada, and Europe. He is also actively engaged in the computational biology community, having organized RECOMB 2011 in Vancouver, BC, chairing the program committee of RECOMB.